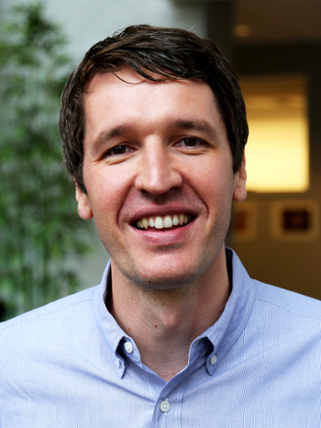
Tell me about your work on child opportunity.
I’m interested in how neighborhood contexts shape children’s healthy development. At DDK, we have about 1.5 billion estimates, including the Child Opportunity Index (COI), which provides neighborhood-level measurements of 29 key factors, such as good schools, green spaces and health care access, which are necessary for healthy child development.It is a big challenge to produce this data on an industrial scale in a way that is robust, replicable and uses the best available approaches to calculate indicators and accurate margins of error.
I’m proud of the work we’ve been doing with users of the COI. Sometimes users don’t have organizational capability to take full advantage of the data. We try to help and also develop efficient processes so that we can assist many users who may have very little staff support.
For example, our users are deciding where to offer certain resources, like where to place early child-hood education centers, where to target hospital resources for preventive care, or which neighbor-hoods to send participants of a housing voucher program. In a lot of those circumstances, it was the COI that made people understand spatial and racial or ethnic inequalities, and helped users to incorporate equity considerations front and center into their decision making.
How are you using machine learning in your work?
I’m working with PhD student Yu-Han Chen on using machine learning to predict infant mortality. Birth is such an interesting point: Virtually all mothers and infants come into contact with the health care system and data are collected, but it’s not being used as much as it could to inform policies to address inequity. Infant mortality is still substantially higher before the first birthday in the U.S. than in other developed countries, and it’s even higher for infants born to African American and Native American mothers.Machine learning is a new technology for the social sciences. A lot of our work is focused on understanding the effects of an intervention or policy change on children’s outcomes, but using machine learning, you can predict the likelihood of something happening. You can optimize certain processes, like the allocation of scarce resources in a costly neonatal or home-visiting program. We use a data set of all registered births in the U.S. It’s comprehensive — there’s no bias in terms of who is in the sample, which is important for equity. We use over 100 variables measured at birth about pregnancy, birth, mothers and delivery, and we use relatively sophisticated algorithms to make a prediction that there will be an adverse outcome. The risk scores obtained from such models could be used to recommend a referral after discharge.
How did you become interested in working with Dean David Weil on wage norms?
My PhD and postdoc were on labor market issues, so this was a good fit. Wages in the low-wage labor market have stagnated or declined in real terms over the last 30 to 40 years. The federal minimum wage is still at $7.25. What can you do to boost wages, especially for people of color and women who are overrepresented in the low-wage sector.I suppose things started with the “Fight for 15” campaign for the $15 minimum wage. More recently, employers started publicly announcing wage increases, most notably Amazon, which raised its minimum wage to $15.
Our study found that higher minimum wages set by companies like Amazon, Walmart and Target lead to higher pay for low-wage workers at nearby businesses. This illustrates a “contagion effect”: When you push one, others follow. From a policy perspective, it shows that movements like the “Fight for 15” have an effect.
It also shows that companies underpay their workers systematically. How else can you explain that all of a sudden Amazon can increase their wages substantially? It’s not that workers are suddenly more productive. You assume that workers are paid as much as they contribute — if they were paid too little they would leave, or if they were paid too much the employer would go out of business. But workers have very little power and employers know how to exploit that.